A pilot study of a novel therapeutic approach to obesity: CNS modification by N.I.R. H.E.G. neurofeedback
11/6/2019
1. Introduction
The endeavor to overcome the obesity epidemic seems Sisyphean now more than ever: weight loss programs fail to produce weight maintenance in the majority of people and while bariatric surgery seems relatively effective for properly selected candidates, it is radical in the sense of irreversibly disrupting a physiologically intact digestive system. A growing amount of literature has highlighted
brain processes involved in eating behavior and indicates that treating obesity cannot remain “brainless” [1-4]. Despite the fact that brain pathways involved in eating behavior have been well
mapped, no treatment aimed at modulating eating dysregulation from its neurocognitive root has been established yet [5-7]. Neurofeedback frameworks induce changes in emotions and in cognitive performance through selective modulation of brain neural activity. Real time functional Magnetic Resonance Imaging (fMRI) and Electroencephalogram (EEG) are well validated technologies anchored in the obesity research. Tools for selective brain activity modification have been explored, with only minimal pioneering research aimed at obesity treatment [5,8]. Near Infra-red Hemoencephalography (N.I.R. H.E.G.) is a different modality, well explored and validated in treating attention deficit hyperactivity disorder (A.D.H.D.) and addiction [9-11] but has not been utilized to date in the obesity field. N.I.R. H.E.G. has unique characteristics which make it especially appealing as a therapeutic brain modulation device for the treatment and prevention of obesity: first, it is a compact, mobile and relatively inexpensive training method, and second, it is specifically designed to target prefrontal areas and practice executive functions which are central to eating modulation. We compared eating behavior, weight and brain activity related to eating control and self-inhibition before and after a
neurofeedback training series in a cohort of 6 healthy overweight to obese young men with overweight to mild obesity aiming to evaluate neurofeedback training with N.I.R. - H.E.G. as a therapeutic
platform for obesity.
2. Methods
Study design: a single arm longitudinal study.
2.1. Participants
We recruited young healthy male participants in the weight range of overweight to mild obesity using poster advertisements in our medical center. We targeted this weight category assuming a potentially reversible dysregulation in relevant brain circuits as opposed to people with severe obesity, in which food related brain activity and hormonal milieu are expected to be long standing and more resistant to modification. Inclusion criteria were: physically and psychiatrically healthy,right-handed, age 20-45, BMI in the range of 28-35 kg/m2.Exclusion criteria: current or past history of any major psychiatric
disorder, major medical or neurological disorders, exposure to drugs likely to influence cerebral blood flow or neurological function within 3 weeks, history of drug or alcohol abuse and general MRI exclusion criteria.
2.2. Intervention
Participants underwent psychological interview and fMRI scans before and after 5 weeks eurofeedback training as described below. Whereas conventional weight loss program team s address the multidisciplinary nature of eating behavior, and include dietitians, psychologists and nurses, we intentionally chose to avoid a directed dietetic consultation in order to isolate the pure effect of
N.I.R. H.E.G. training on eating behavior.
2.3. Neurofeedback
2.3.1. Technology
N.I.R. H.E.G. is a non-invasive neuroimaging device that measures changes in the concentration of oxygenated-hemoglobin (O2Hb) and deoxygenated-hemoglobin (HHb) in cortical blood vessels. Neurofeedback facilitates selective modulation of brain activity which affects cognitive and emotional performance. It has been used successfully in recent years to treat various conditions, including ADHD, Post-traumatic stress disorder and chronic pain [9-14]. This technology is based on the principle of neurovascular coupling: changes in neural activity that are mirrored by changes in blood oxygenation in the region of the activated cortical area (i.e. the increase in O2Hb and the decrease in HHb). NIRS signal is based on the changes in the intrinsic optical absorption of both HHb and O2Hb [15]. The N.I.R. H.E.G. shines alternating red (660 nm) and near infra-red (850 nm) light on a specified area of the brain, through the skull, which is largely translucent to these light wavelengths. Photoelectric cells in a spectrophotometer device worn on the forehead measure the amount of each wavelength. The red/infrared ratio is processed by a computer and translates into a visual signal on a graphical interface. In H.E.G. neurofeedback sessions, participants are given an introspective task, such as relaxation, mindfulness or specific mental focus and they receive continuous visual feedback to guide them towards persistent intentional control of regional brain activity. We used N.I.R. H.E.G. Headband by Biocomp research L.A. The detection probes were placed on the Fp1 and Fp2, and the emission probes were 3 cm lateral from the detection probes along the T3-Fpz-T4 line, according to the international 10/20 system used in electroencephalography.
2.3.2. Neurofeedback sessions
Participants went through 10 H.E.G. sessions that were scheduled to consistent afternoon hours, at their convenience, twice weekly, over 5 weeks of intervention. They were instructed to come to sessions in a “half-satiated” condition in order to focus on hedonic/emotional brain circuits involved in eating behavior rather than physiological circuits, based on the “real”, physiological hunger. The first introductory session lasted 45 min, including orientation and adaptation to the interactive equipment concept. The following sessions lasted 30 min. Each session included 5 min of mindfulness practice while sampling midline fpz point with no feedback and then 10 min of neurofeedback training to each side: fp1 and fp2. Participants were given introspective tasks of mindfulness, increased sense of self-control and focus. These tasks have been previously described to be associated with functions of the superior orbitofrontal cortex (sOFC) and anterior cingulate cortex (ACC) bilaterally [4,6,16,17]. Successive feedback regarding changes in activity in these regions was given analogically with a video imitating the view from an airplane cockpit, where enhanced oxygenated blood flow to frontal areas induced acceleration in flight speed and vice versa. The compliance to HEG training was very good. One participant expressed doubts and required more technical and scientific explanations than the rest of participants.
2.4. Outcome measures
2.4.1. Baseline questionnaire
Prior to intervention, our group's psychologist conducted a semi structured interviewthat included questions addressing chronology of weight gain and loss: childhood weight, age of significant weight
gain, number of previous diets, weight gain attribution and regular eating patterns. Then, participants filled a questionnaire that was built for this study based on eating behavior literature [18-24]. This
questionnaire addressed a spectrum of eating behavior traits rather than diet composition: hunger and satiety awareness, cravings, impulsivity, binge eating, emotional eating, hedonic eating and externally driven eating, mindless eating and self-efficacy assessments of ability to change. Participants also completed the Center for Epidemiologic Studies Depression Scale (CESD) [25]. We repeated the questionnaire 6 months after the training. The variables used for the current data analysis were as follows: Overeating e a single item x-point Likert scale assessing eating beyond feeling full ranging from “never” to “most of the time”. External eating e four X-point Likert scale items assessing external triggers to eating (not physical hunger), such as eating more because food tasted or smelled good, eating because others were eating or seeing food. The range was from “never” to “always”.
Emotional eating e eating in reaction to emotional states (anger, stress, worry, etc.) as triggers to eating. The X-point scale ranged from “never” to “always”.
2.5. Anthropomorphic measurements
Height, weight and BMI were measured before intervention, at week 6, soon after accomplishing the Neurofeedback sessions series and 6 months later (weight reported by phone).
2.6. Functional MRI sessions
2.6.1. Experimental paradigm
2.6.1.1. Structural MRI acquisition.
For anatomical localization of functional data and 3D rendering of the cortical surface, high resolution (1 mm3, Matrix 256 _ 256, FOV 25.6 cm) images of the entire brain were acquired for each subject, using a standard 3D inversion recovery prepared FSPGR T1 weighted sequence: TR ¼ 7.3 s; TE ¼ 2.7; flip angle ¼ 20_; TI ¼ 450 ms. 2.6.1.2. fMRI data acquisition. Functional scanning was performed using a 3 T whole body MRI system (GE Signa HDxt, v16VO2) equipped with 8-channel head coil. Each scan was acquired using T2*-weighted gradient-echo echo-planar images (GE-EPI): TR ¼ 2.5 s; TE ¼ 35 ms; flip angle ¼ 81; matrix size 64 _ 64, FOV 20 _ 20 cm. Thirty-five contiguous oblique axial slices covering the whole brain (slice width 3 mm, 0.3 mm gap) voxel size ¼ 3.125 mm _ 3.125 mm _ 3.3 mm fMRI paradigms were presented using BrainWave 2.0 (GE Healthcare, Inc). Visual stimuli were back-projected by a radio frequency shielded projector system and viewed through a mirror device. Responses were recorded using a Lumina response box (Lumina, Cedrus Corporation, CA, USA).
2.6.1.3. fMRI task. Rapid, event-related task with a total of 480 trials, 360 Go and 120 No Go trials; thus, the probability of NoGo trials was 25%. Letters were projected in sequence (presentation
time: 1250 ms). Go and NoGo trials were randomly interspersed throughout the whole 6-minute task. Participants were instructed to focus on the screen on which the words would appear. They were
told to press a button with their right index finger in response to the word “PRESS” presented in green on the screen and not to press the button for the word “PRESS” presented in red, which was the
No-Go stimulus. Prior to scanning, each participant underwent a training session in which they practiced the task outside the scanner to insure that they understood the task. The experimental task was programmed using Presentation software (Version 18.0, Neurobehavioral Systems, Inc., Berkeley, CA, www.neurobs.com).
2.6.2. Standardized satiety status during fMRI scanning
For our specific modulation goal, the suitable in-scanner satiety status was determined as “half satiated”. On one hand, hunger activates brain circuits involved in homeostatic appetite while, on the other hand, complete satiety will shut down any food craving. For that purpose, participants were instructed to come to sessions neither hungry nor extremely full. They were advised to have a medium to large meal 1e2 h before sessions. We documented the last meal (timing, content, estimated caloric value) and recorded subjective estimates of participants' hunger, satiety, fullness and prospective eating using visual analog scales.
2.6.2.1. fMRI data processing.
Preprocessing and statistical analyses of functional images were performed using SPM12 (www.fil.ion.ucl.ac.uk/spm). The first five dummy scans were discarded to avoid artefacts due to magnetic saturation effects and the remaining images were preprocessed implementing the following steps: slice time correction to the first slice; realignment of scans to the middle image to correct for head motion; coregistration to the mean functional image and segmentation of the anatomical image with the new segment algorithm; normalization to the Montreal Neurological Institute (MNI) space using SPM's DARTEL toolbox; smoothing with an 8-mm Gaussian kernel (full width at half maximum). The preprocessed images were then analyzed using the general linear model (GLM) convolving the correct responses for each type of stimuli with the canonical hemodynamic response function. Incorrect responses were not used for computing contrasts since there were not enough incorrect responses to have sufficient power. Additionally, the GLM included six movement parameters as regressors of no interest. Finally, for the first-level analyses we computed for each subject, contrast images using the subtraction of correct ‘‘no-go’’- ‘‘go’’ trials (correct inhibition- hit). Individual contrast images were entered in to a second-level analysis, using a fixed-effect analysis for pre- and post- intervention respectively.
2.7. Statistical methods
Average change from before to after intervention of study outcomes was tabulated and tested with paired t test. One-tail test was carried out just to obtain indication in this small study. This is a pilot study and formal power analysis was not required. Also the percent change of weight over time was plot overlaid with the average change to illustrate the trend over time of each study participant.
Due to the small sample-size we did not conduct a two-sample analysis between the post and pre fMRI sessions, but rather first level images were entered into two fixed-effects one-sample-analyses
separately, in order to examine whether there is a certain trend between -the pre and post conditions. All analyses were tested at an un-corrected significance level of p < 0.001, k > 10.
3. Results
Out of 9 candidates 3 did not meet criteria or could not commit to the demands of the study therefore we recruited 6 participants. Baseline demographic and physical characteristics are described in Table 1.
3.1. Baseline weight and eating behavior.
All participants were at peak weight when the study started, averaging 98.8 kg with an average BMI of 32 kg/m2, four of the six had past weight loss attempts. The average CES-D score was 6.0 (SD ¼ 5.1), which indicated the absence of depression in this sample. None of the participants met criteria for binge eating disorder or night eating. Their average score on the overeating item was 2.7 (SD ¼ 0.52, range 0-3). On the composite external eating measure, the average was 8.8 (SD ¼ 1.94, range 0e16). The emotional eating average was 9.8 (SD ¼ 5.38, range 0-44). The participants had relatively high levels of self-efficacy: their anticipated ability to change eating habits averaged 3.8 (SD ¼ 1, range 0-5) and changing exercise habits averaged 3.5 (SD ¼ 0.84, range 0-5).
3.2. Behavioral changes
Six months post intervention, participants reported significant changes in their eating behaviors, mainly reduction in overeating, external eating and emotional eating. External Eating Score decreased significantly with one-tail paired-t test with p ¼ 0.0421. (See Fig. 1).
3.3. Changes in weight
After the 5 week training, the average weight was 99.25 kg (SD ¼ 8.67), an insignificant increase from baseline. Six months post intervention, participants lost significant weight to an average
of 96 kg (SD ¼ 7.95). Weight reduction reached statistical significance with one-tail paired-t test (p Value ¼ 0.01). (See Fig. 2).
3.4. fMRI results
3.4.1. Pre-intervention
The (correct inhibition- hit) contrast yielded activation in the right temporal inferior area [x, y, z ¼ _18, _40, 47 (BA 20)], right fusiform area [x, y, z ¼ 42, _52, _13 (BA 37)], right frontal superior area [x, y, z ¼ 21, _10, 59 (BA 6)] and right frontal inferior area [x, y, z ¼ 48,11, 29 (BA 9)] at a significant level of 0.001 with a cluster size of 10 (see Table 2).
3.4.2. Post-intervention
The (correct inhibition- hit) contrast yielded activation in the right frontal inferior area [x, y, z ¼ 48,11, 32 (BA 9)], in the left insula [x, y, z ¼ _36, 26, 2 (BA 47)] and in the right parietal superior area [x, y, z ¼ 33, _55, 62 (BA 7)] at a significant level of 0.001 with a cluster size of 10 (see Fig. 3 right panel, Table 2).
4. Discussion
A series of N.I.R. H.E.G. neurofeedback sessions produced a statistically significant weight loss, a trend of behavioral improvement in external eating and self-control and enhanced activity and volume in the OFC in fMRI in a cohort of 6 healthy overweight and obese male participants. In a recent study, 8 obese subjects were successfully trained to up-regulate functional connectivity between the dorsolateral prefrontal cortex (dlPFC) and the ventromedial prefrontal cortex (vmPFC) during rt-fMRI series of 4 days, 3 neurofeedback sessions per day, with a trend towards less high-calorie food choices [5]. This study correlates with emerging evidence of cortical plasticity and supports our assumption that neurofeedback induced modulation of prefrontal areas has a therapeutic potential for eating behavior and obesity. While using a different neurofeedback approach and goals, we chose to correlate brain changes with more extensive aspects of eating behavior and longer term follow-up of these changes and weight dynamics. Findings of this proof-of-concept study indicate that overweight and obese participants can enhance their orbitofrontal activity after series of neurofeedback sessions with correlating increased self-inhibition and weight loss. Functional MRI and behavioral changes were observed after 10 N.I.R. H.E.G. neurofeedback sessions, despite the deliberate absence of eating related context during sessions. We chose to explore whether “context-neutral” training of the OFC will lead to improvement in self-control and eating behavior, regarding them as part of a whole spectrum of executive functions. In addition, our findings suggest that a 10-session series yields effects that last for 6 months and could have been augmented through additional maintenance sessions, for example, once a month after completing the primary series. N.I.R.H.E.G. is a less explored neurofeedback modality compared to E.E.G. and rt-fMRI based neurofeedback. This modality has unique characteristics which makes it especially appealing as a therapeutic brain modulation device for the treatment and prevention of obesity: It is a compact, mobile and relatively low-cost neurofeedback modality with an inherent focus on frontal cortical areas. Its short penetrance through the skull and low spatial resolution (1 cm) and hence, limitation to cortical areas, were negligible in our study, since the prefrontal cortex is a pivotal target for modulation and spatial resolution during the training wasn't critical since we used fMRI to evaluate neural changes. The average education level of our participants was relatively high, probably resulting from the recruitment method using advertisements in an academic hospital. We believe this characteristic does not preclude good compliance and training results among less educated populations since neurofeedback is widely used in children with ADHD with well documented benefits. The main weaknesses of our study are the lack of no-treatment control group, the small sample size, allowing our results to only show a trend without a statistical power and the inaccessible numerical data accumulated during the neurofeedback training sessions due to specific equipment limitations, preventing the option to evaluate dynamics in brain activation during sessions and to compare between sessions. The study questionnaires were intended to characterize eating behavior before and after intervention. We acknowledge that an additional dietary questionnaire would have added important data regarding intentional expressions of these traits.
We find the results of our study encouraging towards further exploration in a larger scale randomized controlled study parallel to technological enhancement of compact and feasible neurofeedback
modalities. The ability to train and improve self-control and other executive functions can potentiate a change in eating behaviors as part of comprehensive treatment and prevention for obesity.
NCT02480179.
Conflict of interest: None.
References
[1] Michaelides M, Thanos PK, Volkow ND, Wang G-J. Functional neuroimaging in obesity. Psychiatr Ann 2011;41:496e500.
[2] Val-Laillet D, Aarts E, Weber B, Ferrari M, Quaresima V, Stoeckel LE, et al. Neuroimaging and neuromodulation approaches to study eating behavior and prevent and treat eating disorders and obesity. NeuroImage Clin 2015;8:1e31.
[3] Suzuki K, Jayasena CN, Bloom SR. Obesity and appetite control. Exp Diabetes Res 2012;2012.
[4] Batterink L, Yokum S, Stice E. Body mass correlates inversely with inhibitory control in response to food among adolescent girls: an fMRI study. Neuroimage 2010;52:1696e703.
[5] Spetter MS, Malekshahi R, Birbaumer N, Lührs M, van der Veer AH, Scheffler K, et al. Volitional regulation of brain responses to food stimuli in overweight and obese subjects: a real-time fMRI feedback study. Appetite 2017;112: 188e95.
[6] Carnell S, Gibson C, Benson L, Ochner CN, Geliebter A. Neuroimaging and obesity: current knowledge and future directions. Obes Rev 2012;13:43e56.
[7] Hanlon CA, Hartwell KJ, Canterberry M, Li X, Owens M, Lematty T, et al. Reduction of cue-induced craving through realtime neurofeedback in nicotine users: the role of region of interest selection and multiple visits. Psychiatry Res Neuroimaging 2013;213:79e81.
[8] Ihssen N, Sokunbi MO, Lawrence AD, Lawrence NS, Linden DEJ. Neurofeedback of visual food cue reactivity: a potential avenue to alter incentive sensitization and craving. Brain Imaging Behav 2017;11:915e24.
[9] Birbaumer N, Ramos Murguialday A, Weber C, Montoya P. Neurofeedback and brain-computer interface clinical applications. Int Rev Neurobiol 2009;86: 107e17.
[10] Arns M, Heinrich H, Strehl U. Evaluation of neurofeedback in ADHD: the long and winding road. Biol Psychol 2014;95:108e15.
[11] Mize W. Hemoencephalographyea new therapy for attention deficit hyperactivity disorder (ADHD): case report. J Neurother 2004;8:77e97.
[12] Hassan MA, Fraser M, Conway BA, Allan DB, Vuckovic A. The mechanism of neurofeedback training for treatment of central neuropathic pain in paraplegia: a pilot study. BMC Neurol 2015;15:1e13.
[13] Fragedakis TM, Toriello P. The development and experience of combat-related PTSD: a demand for neurofeedback as an effective form of treatment. J Couns Dev 2014;92:481e8.
[14] Niv S. Clinical efficacy and potential mechanisms of neurofeedback. Pers Indiv Differ 2013;54:676e86.
[15] Steinbrink J, Villringer A, Kempf F, Haux D, Boden S, Obrig H. Illuminating the BOLD signal: combined fMRI-fNIRS studies. Magn Reson Imaging 2006;24: 495e505.
[16] Beaver JD, Lawrence AD, Van Ditzhuijzen J, Davis MH, Woods A, Calder AJ. behavioral/systems/cognitive individual differences in reward drive predict
neural responses to images of food. J Neurosci 2006;26:5160e6.
[17] Rich EL, Wallis JD. Decoding subjective decisions from orbitofrontal cortex. Nat Neurosci 2016;19:973e80.
[18] Cepeda-Benito A, Fernandez MC, Moreno S. Relationship of gender and eating disorder symptoms to reported cravings for food: construct validation of state and trait craving questionnaires in Spanish. Appetite 2003;40:47e54.
[19] Moreno S, Rodríguez S, Fernandez MC, Tamez J, Cepeda-Benito A. Clinical validation of the trait and state versions of the food craving questionnaire. Assessment 2008;15:375e87. Patton JH, Stanford MS, Barratt ES. Factor structure of the barratt impulsiveness scale. J Clin Psychol 1995;51:768e74.
[21] Richards JB, Zhang L, Mitchell SH, de Wit H. Delay or probability discounting in a model of impulsive behavior: effect of alcohol. J Exp Anal Behav 1999;71: 121e43.
[22] Monterosso J, Ainslie G. Beyond discounting: possible experimental models of impulse control. Psychopharmacology (Berl) 1999;146:339e47.
[23] Lowe MR, Butryn ML, Didie ER, et al. The Power of Food Scale. A new measure of the psychological influence of the food environment. Appetite 2009;53: 114e8.
[24] Van Strien T, Rookus MA, Bergers GP, Frijters JE, Defares PB. Life events, emotional eating and change in body mass index. Int J Obes 1986;10:29e35.
[25] Radloff LS. The CES-D scale: a self report depression scale for research in the general. Appl Psychol Meas 1977;1:385e401.
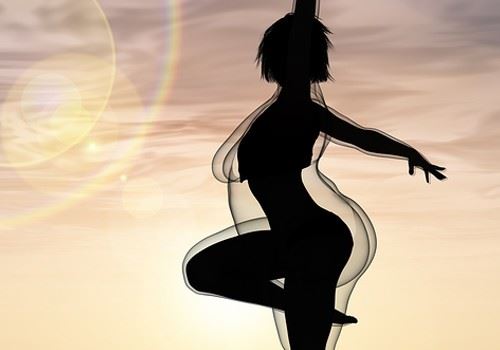